Run a Local LLM with LM Studio
Run Llama|Mistral|Phi|Gemma|DeepSeek|Qwen 2.5 locally on your computer!
Picture this: your own AI buddy, running right on your computer, ready to chat, code, or solve problems—no internet, no fees, just you and your machine. That’s what running a Large Language Model (LLM) locally offers, and with LM Studio, it’s simpler than you’d think.
Introduction
So I have been tinkering with AI and I wanted to set up a LLM model such as DeepSeek-R1 locally. I came across LM Studio which allowed me run an AI model fully hosted on my PC within 10 minutes. Pretty amazing! I am using my mini PC UM780 XTX with AMD Ryzen 7 7840HS with 32GB of RAM.
This guide will show you how to set up an LLM using LM Studio. I’ll also highlight some popular CPU setups to match your hardware and throw in a quick comparison of top models—including the DeepSeek-R1 distilled series. Let’s get started!
Why Go Local with an LLM?
Running an LLM on your device means privacy, no subscription costs, and offline access. Plus, you can play with open-source models like a kid in a candy store and it is pretty cool!
What’s LM Studio?
LM Studio is a free, all-in-one app for Windows, macOS, and Linux that lets you run LLMs without diving into code (unless you want to). It grabs models from Hugging Face, offers a chat interface, and can even mimic OpenAI’s API locally. It’s optimised for your CPU, making it perfect for folks like me with a Ryzen 7 and no NVIDIA GPU.
Step-by-Step: Running an LLM with LM Studio
Here’s the playbook—I’ve tested it myself to keep it spot-on and straightforward.
1. Check Your Gear
Your computer needs to be up to the task. You can check the full system requirements here but here’s the baseline:
- CPU: Modern, with AVX2 support (e.g., Intel Core i5 or AMD Ryzen 5 from the last 8-10 years) or any Apple Silicon (M1-M4—macOS-ready).
- RAM: 16GB minimum for small models; 32GB+ is ideal for wiggle room.
- Storage: SSD with 10-20GB free (models vary from a few GB to tens of GB).
- GPU (Optional): NVIDIA with CUDA speeds things up, but CPU-only works fine—my Radeon 780M sits this one out 😢.
I’ll list some CPU configs later as reference to size up your rig.
2. Grab LM Studio
Head to lmstudio.ai, download the ~400MB installer for your OS, and run it. Follow the steps, launch the app, and you’re in.
3. Choose and Download a Model
Open LM Studio and hit the “Discover” tab (magnifying glass icon). It’ll suggest models your system can handle. Here are some stars to pick from:
- Mistral 7B: Fast and flexible for everyday tasks (~8-10GB RAM, quantized).
- LLaMA 3.1 8B: A powerhouse for chat and reasoning (~10-12GB RAM).
- DeepSeek-R1-Distill-Qwen-7B: A distilled gem for math and logic (~8-12GB RAM).
- Phi-3 Mini (3.8B): Tiny but mighty, perfect for light setups (~5-6GB RAM).
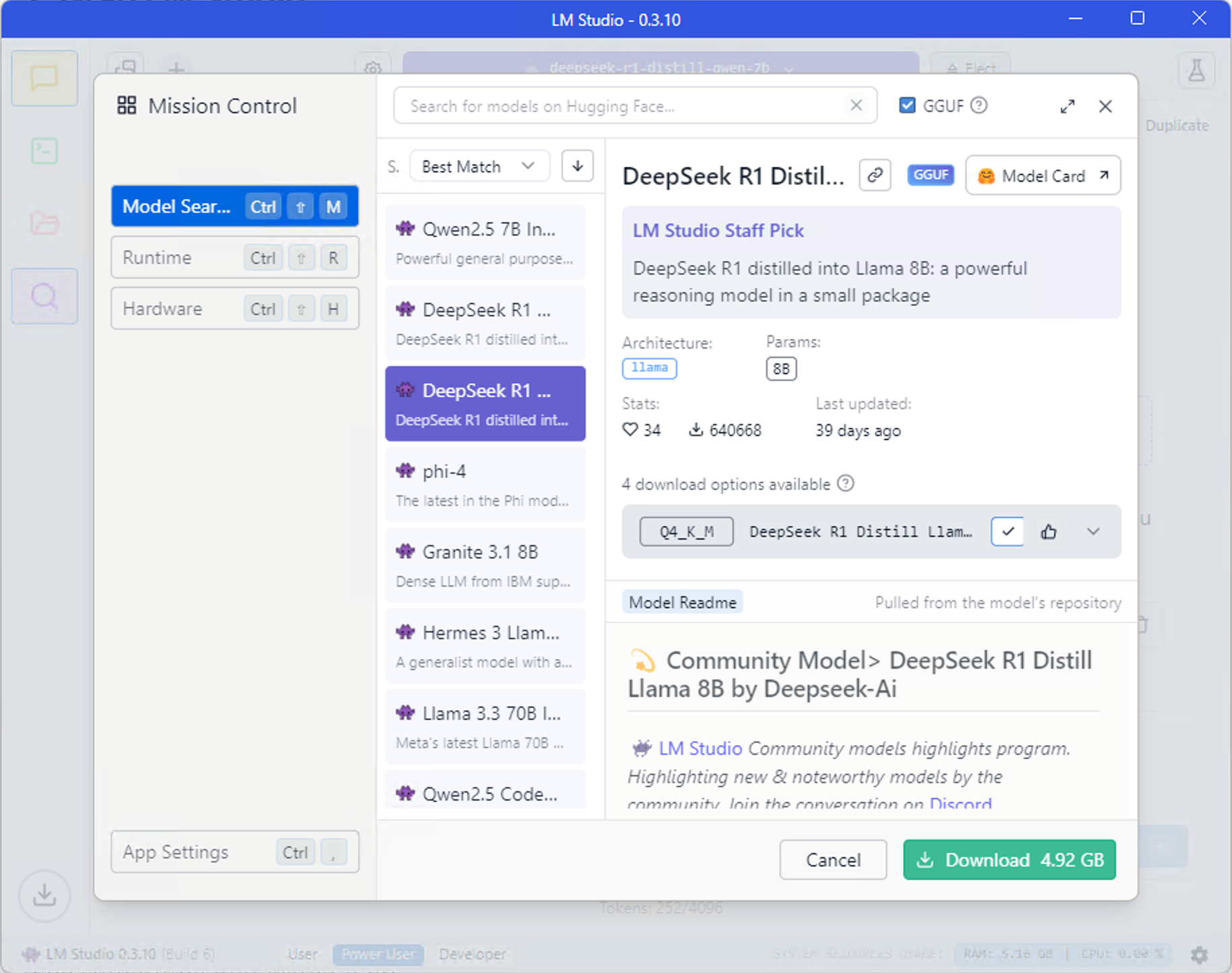
Click a model, pick a quantized version (like “Q4_K_M” to save memory), and hit “Download.” Bigger models take a bit, so maybe make some 🍵.
4. Load It Up and Chat
Switch to the “Chat” tab (speech bubble), select your model from the dropdown, and click “Load.” Your CPU will buzz for a sec as it loads into RAM. Once it’s ready, type something and watch it go.
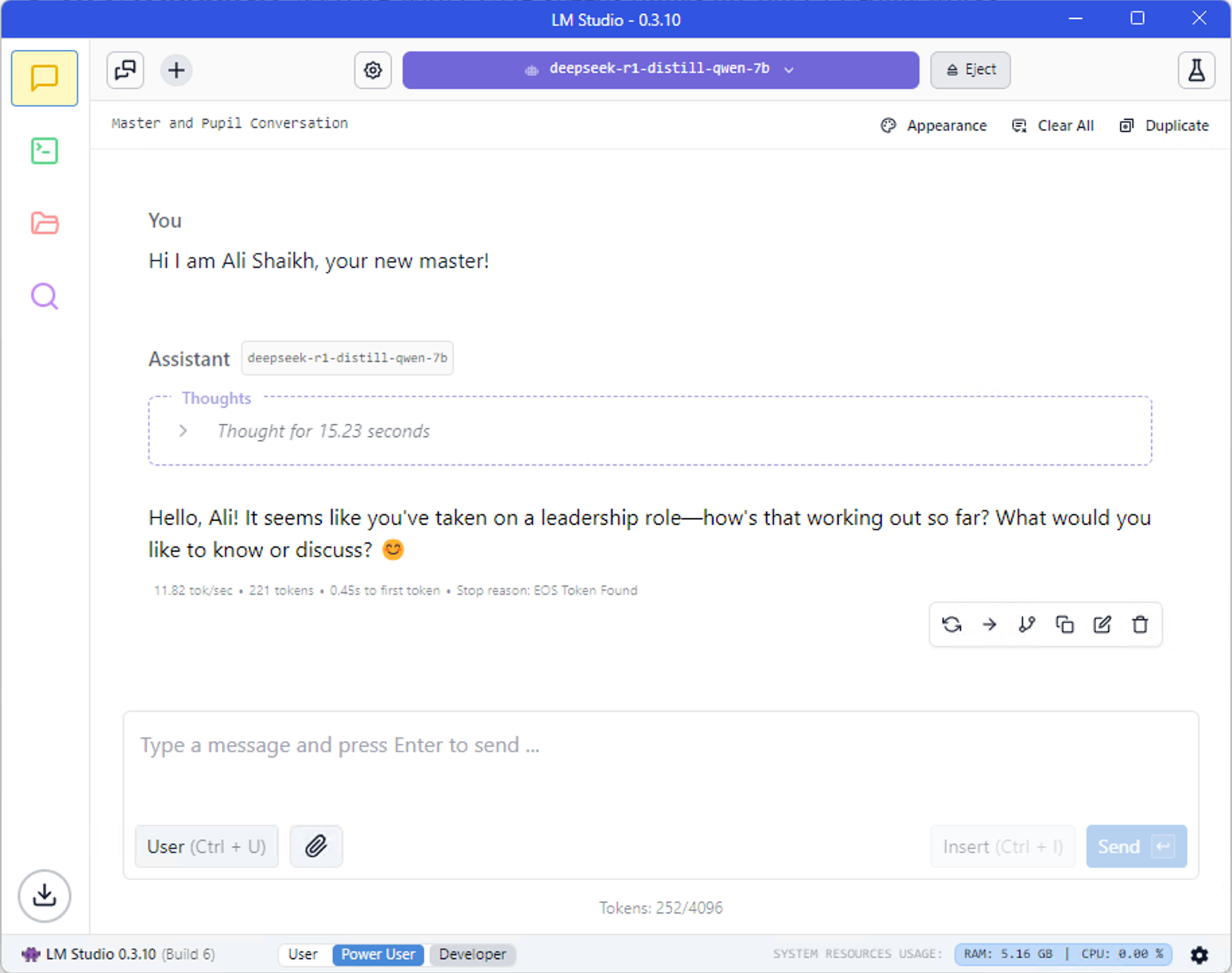
6. Have Fun!
Boom—you’ve got an LLM at your command, offline and private. Test prompts, swap models, or use it to brainstorm. It’s your AI playground.
Comparing the Models
Not sure which model to choose? Here’s a quick rundown of my top picks, including DeepSeek-R1 distilled, based on size, speed, and smarts:
Model | Size | RAM | Speed | Best For |
---|---|---|---|---|
Mistral 7B | 7B | 8-10GB | 8-12 t/s | Writing, Q&A |
LLaMA 3.1 8B | 8B | 10-12GB | 5-10 t/s | Chat, Reasoning |
DeepSeek-R1 (Qwen-7B) | 7B | 8-12GB | 6-12 t/s | Math, Coding |
Phi-3 Mini | 3.8B | 5-6GB | 10-15 t/s | Light Tasks |
Winner? If you’re into reasoning or coding, grab DeepSeek-R1-Distill-Qwen-7B. For everyday chatting, LLaMA 3.1 8B is your pal. Mistral’s a speed demon, and Phi-3’s the lightweight champ.
Popular CPU Configurations and What They Can Run
Your CPU and RAM set the stage. Here’s what common setups can handle, checked against real benchmarks:
Entry-Level: Intel Core i5-12400 / AMD Ryzen 5 5600X / Apple M1 + 16GB RAM
- Cores/Threads: 6/12 (Intel/AMD), 8/8 (M1)
- Models: Phi-3 Mini, Mistral 7B (quantized)
- Performance: ~8-12 tokens/sec for 7B
- Notes: M1’s efficiency rocks; 16GB caps bigger models.
Mid-Range: Intel Core i7-12700 / AMD Ryzen 7 5800X / Apple M2 + 32GB RAM
- Cores/Threads: 8/16 (Ryzen), 12/20 (Intel), 8/10 (M2)
- Models: Mistral 7B, LLaMA 3.1 8B, DeepSeek-R1-Distill-Qwen-7B, Qwen-14B
- Performance: ~5-10 tokens/sec for 7B-14B
- Notes: M2’s neural engine kicks in; my Ryzen 7 7840HS (8/16) fits here—8B models fly.
High-End: Intel Core i9-13900K / AMD Ryzen 9 7950X / Apple M3 Max or M4 + 64GB RAM
- Cores/Threads: 16-24/32 (Intel/AMD), 14/14 (M3 Max), 10-12/10-12 (M4 base)
- Models: LLaMA 3.1 13B, DeepSeek-R1-Distill-Qwen-32B
- Performance: ~3-8 tokens/sec for 13B-32B
- Notes: M3 Max and M4 (10-12 cores) soar with macOS tweaks; 64GB tackles big models easy.
Tips to Nail It
- Quantize Smart: Use 4-bit models (e.g., Q4_K_M) to fit more in RAM—they’re still sharp.
- Free Up Space: Shut down Chrome or games to give your LLM breathing room.
- Mix and Match: Download a few models—DeepSeek-R1 for logic, LLaMA for chats.
- Troubleshoot: If it lags or crashes, check LM Studio’s logs for hints.
Final Thoughts
So this is how you run a LLM locally with LM Studio, it's SUPER COOL!!. With my Ryzen 7 and 32GB RAM, I’m loving DeepSeek-R1-Distill-Qwen-7B and LLaMA 3.1 8B.
Grab LM Studio and give DeepSeek-R1 a spin on your rig—I’d love to hear how it goes in the comments!
Member discussion